TF-JOB
이전에 올렸던 글 HyperparameterTuning(Katib)에서는 Experiment를 생성한 뒤 그안에서 여러 Trials들이 서로 통신하면서 Hyperparameter를 생성하는 것에 대하여 설명하였습니다.
이러한 Katib는 TF-JOB을 서로 통신하면서 Hyperparameter를 Tuning한다고 할 수 있습니다.
즉, Kubeflow에서는 하나의 Model을 Trainning을 하기위한 방법을 제공한다는 것 입니다.
이번 글에서는 Tensorflow의 Trainning을 위한 TF-JOB에 대하여 설명합니다.
사전사항
HyperparameterTuning(Katib)에서 Build하여 올린 Image
참고사항
이번 글에서는 Tensorflow에서 Trainning을 하기위한 TF-JOB에 대해서만 작성합니다. 다른 Framework는 옆의 링크를 참조하시길 바랍니다. Kubeflow-Trainning
TF-JOB 이란?
TF-JOB이란 Tensorflow Model을 Kubeflow안에서 Trainning할 수 있게 도와주는 Kubeflow의 기능 중 하나입니다.
Kubernetes의 다른 작업과 마찬가지로 .yaml File을 작성하여 Deployment합니다.
먼저 TF-JOB은 지정한 개수만큼의 POD으로서 동작하므로 사전에 PVC와 PV를 작성하여 적용시켜야 합니다.
내부적으로 자동적으로 생성하는 것이 아니므로 어떠한 Version에서도 동일한 작업입니다.
1. PV 작성 및 apply
#tfjob-cnn-pv.yaml
apiVersion: v1
kind: PersistentVolume
metadata:
name: tfevent-volume
labels:
type: local
app: tfjob
spec:
capacity:
storage: 10Gi
storageClassName: standard
accessModes:
- ReadWriteMany
hostPath:
path: /home/jyhwang/kubeflow/tf-job/tfjob-storage
2. PVC 작성 및 apply
#tfjob-cnn-pvc.yaml
apiVersion: v1
kind: PersistentVolumeClaim
metadata:
name: tfevent-volume
namespace: kubeflow
labels:
type: local
app: tfjob
spec:
accessModes:
- ReadWriteMany
resources:
requests:
storage: 10Gi
3. TF-JOB 생성 및 apply
#tfjob-cnn.yaml
apiVersion: "kubeflow.org/v1"
kind: "TFJob"
metadata:
name: "tfjob-cnn"
namespace: kubeflow
spec:
cleanPodPolicy: None
tfReplicaSpecs:
Worker:
replicas: 3
restartPolicy: Never
template:
spec:
containers:
- name: tensorflow
image: wjddyd66/cnn-example:15.0
command:
- "python"
- "/var/tf_cnn/cnn-example.py"
- "---learning_rate=0.01"
- "--num_epoch=1000"
volumeMounts:
- mountPath: "/train"
name: "training"
volumes:
- name: "training"
persistentVolumeClaim:
claimName: "tfevent-volume"
주의 사항
- TF-JOB의 volumes의 PVC이름과 작성한 PVC, PV .yaml FIle의 이름은 동일하여야 합니다.
- Docker Image로 Build한 Image를 반드시 포함하여야 합니다.
- 실질적으로 작업을 하는 Worker에 대한 주요 Parameter는 다음과 같습니다.
- Chief: 실질적으로 Trainning을 분산처리하기위한 orchestrating trainning을 관리합니다.(만약 지정하지 않으면 worker-0가 Chief가 됩니다.)
- Worker: 실질적으로 Trainning을 실행하는 Pod입니다. 위의 .yaml File에서는 3으로 지정하였으므로 worker-0, worker-1, worker-2 로서 Trainning되는 pod이 생성되고 Chief를 지정하지 않았으므로 자동적으로 worker-0이 Chief가 되어 Trainning 됩니다.
- HyperparameterTuning(Katib)와 달리 원본 Code에 Parser를 추가하여도 되고 안해도 됩니다. TF-JOB은 그저 Model을 Trainning하기 때문에 Hyperparameter에 대한 범위를 지정하지 않습니다.
- HyperparameterTuning(Katib)와 달리 Object즉, Goal이 없습니다. 단순한 Trainning이지 어떠한 목적도 가지고 있지 않습니다.
- pv와 pvc를 지정하지 않으면 Pod 자체가 생성되지 않습니다.
확인 결과 다음과 같이 3개의 Pod에서 Trainning 되는 것을 확인할 수 있습니다.
$ kubectl get po -n kubeflow
...
tfjob-cnn-worker-0 0/1 Completed 0 35m
tfjob-cnn-worker-1 0/1 Completed 0 35m
tfjob-cnn-worker-2 0/1 Completed 0 35m
TF-JOB 확인
TF-JOB을 Monitor하고 싶은 경우 다음의 명령어로 실행할 수 있습니다.
kubectl -n kubeflow get tf-job [Job-name] -o yaml
$ kubectl -n kubeflow get tfjob tfjob-cnn -o yaml
apiVersion: kubeflow.org/v1
kind: TFJob
metadata:
annotations:
kubectl.kubernetes.io/last-applied-configuration: |
{"apiVersion":"kubeflow.org/v1","kind":"TFJob","metadata":{"annotations":{},"name":"tfjob-cnn","namespace":"kubeflow"},"spec":{"cleanPodPolicy":"None","tfReplicaSpecs":{"Worker":{"replicas":3,"restartPolicy":"Never","template":{"spec":{"containers":[{"command":["python","/var/tf_cnn/cnn-example.py","---learning_rate=0.01","--num_epoch=1000"],"image":"wjddyd66/cnn-example:15.0","name":"tensorflow","volumeMounts":[{"mountPath":"/train","name":"training"}]}],"volumes":[{"name":"training","persistentVolumeClaim":{"claimName":"tfevent-volume"}}]}}}}}}
creationTimestamp: "2019-11-25T01:28:19Z"
generation: 1
name: tfjob-cnn
namespace: kubeflow
resourceVersion: "22865"
selfLink: /apis/kubeflow.org/v1/namespaces/kubeflow/tfjobs/tfjob-cnn
uid: ff018bb5-7062-4000-b9a9-1a0aea8efcf8
spec:
cleanPodPolicy: None
tfReplicaSpecs:
Worker:
replicas: 3
restartPolicy: Never
template:
spec:
containers:
- command:
- python
- /var/tf_cnn/cnn-example.py
- '---learning_rate=0.01'
- --num_epoch=1000
image: wjddyd66/cnn-example:15.0
name: tensorflow
volumeMounts:
- mountPath: /train
name: training
volumes:
- name: training
persistentVolumeClaim:
claimName: tfevent-volume
status:
completionTime: "2019-11-25T01:50:58Z"
conditions:
- lastTransitionTime: "2019-11-25T01:28:19Z"
lastUpdateTime: "2019-11-25T01:28:19Z"
message: TFJob tfjob-cnn is created.
reason: TFJobCreated
status: "True"
type: Created
- lastTransitionTime: "2019-11-25T01:37:05Z"
lastUpdateTime: "2019-11-25T01:37:05Z"
message: TFJob tfjob-cnn is running.
reason: TFJobRunning
status: "False"
type: Running
- lastTransitionTime: "2019-11-25T01:50:58Z"
lastUpdateTime: "2019-11-25T01:50:58Z"
message: TFJob tfjob-cnn successfully completed.
reason: TFJobSucceeded
status: "True"
type: Succeeded
replicaStatuses:
Worker:
succeeded: 3
startTime: "2019-11-25T01:28:19Z"
생성한 Job을 삭제하기 위해서는 다음의 명령어를 실행합니다.
$ kubectl -n kubeflow delete tfjob [Job-name]
주의 사항
현재 위의 명령어로 인하여 TF-JOB을 삭제하게 되어도 Pod만 사라지지 생성한 PVC나 PV는 삭제되지 않는다.
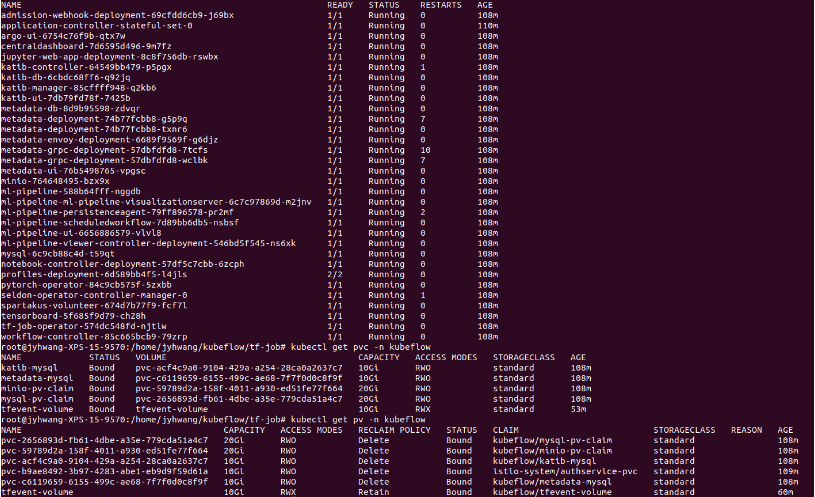
따라서 불필요한 PV와 PVC를 삭제하기 위해서는 강제로 삭제하는 수 밖에 없다.
참고사항(PV, PVC 강제 삭제)
PV나 PVC를 강제로 삭제하기 위해서는 다음과 같은 Option을 추가로 설정한다. --force --grace-period=0
예시
# PVC 삭제
$ kubectl delete pvc -n kubeflow tfevent-volume --force --grace-period=0
# PV 삭제
$ kubectl delete pv -n kubeflow tfevent-volume --force --grace-period=0
TF-JOB의 실질적인 실행을 확인하기 위해서는 LOG를 살펴볼 수 있다.
kubectl logs -n kubeflow [Pod Name]
tfjob-cnn-worker-0을 살펴보면 다음과 같다.
$ kubectl logs -n kubeflow tfjob-cnn-worker-0
WARNING: Logging before flag parsing goes to stderr.
W1125 01:37:06.313838 139694476597056 deprecation_wrapper.py:119] From /var/tf_cnn/cnn-example.py:73: The name tf.placeholder is deprecated. Please use tf.compat.v1.placeholder instead.
Downloading data from https://www.cs.toronto.edu/~kriz/cifar-10-python.tar.gz
170500096/170498071 [==============================] - 236s 1us/step
170508288/170498071 [==============================] - 236s 1us/step
W1125 01:41:07.192519 139694476597056 deprecation_wrapper.py:119] From /var/tf_cnn/cnn-example.py:33: The name tf.truncated_normal is deprecated. Please use tf.random.truncated_normal instead.
W1125 01:41:07.206413 139694476597056 deprecation_wrapper.py:119] From /var/tf_cnn/cnn-example.py:37: The name tf.nn.max_pool is deprecated. Please use tf.nn.max_pool2d instead.
W1125 01:41:07.272838 139694476597056 deprecation.py:506] From /var/tf_cnn/cnn-example.py:64: calling dropout (from tensorflow.python.ops.nn_ops) with keep_prob is deprecated and will be removed in a future version.
Instructions for updating:
Please use `rate` instead of `keep_prob`. Rate should be set to `rate = 1 - keep_prob`.
W1125 01:41:07.298765 139694476597056 deprecation.py:323] From /var/tf_cnn/cnn-example.py:84: softmax_cross_entropy_with_logits (from tensorflow.python.ops.nn_ops) is deprecated and will be removed in a future version.
Instructions for updating:
Future major versions of TensorFlow will allow gradients to flow
into the labels input on backprop by default.
See `tf.nn.softmax_cross_entropy_with_logits_v2`.
W1125 01:41:07.325443 139694476597056 deprecation_wrapper.py:119] From /var/tf_cnn/cnn-example.py:85: The name tf.train.RMSPropOptimizer is deprecated. Please use tf.compat.v1.train.RMSPropOptimizer instead.
W1125 01:41:07.485331 139694476597056 deprecation.py:506] From /usr/local/lib/python2.7/dist-packages/tensorflow/python/training/rmsprop.py:119: calling __init__ (from tensorflow.python.ops.init_ops) with dtype is deprecated and will be removed in a future version.
Instructions for updating:
Call initializer instance with the dtype argument instead of passing it to the constructor
W1125 01:41:07.682564 139694476597056 deprecation_wrapper.py:119] From /var/tf_cnn/cnn-example.py:89: The name tf.summary.scalar is deprecated. Please use tf.compat.v1.summary.scalar instead.
W1125 01:41:07.685440 139694476597056 deprecation_wrapper.py:119] From /var/tf_cnn/cnn-example.py:92: The name tf.summary.merge_all is deprecated. Please use tf.compat.v1.summary.merge_all instead.
W1125 01:41:07.686357 139694476597056 deprecation_wrapper.py:119] From /var/tf_cnn/cnn-example.py:94: The name tf.Session is deprecated. Please use tf.compat.v1.Session instead.
2019-11-25 01:41:07.687160: I tensorflow/core/platform/cpu_feature_guard.cc:142] Your CPU supports instructions that this TensorFlow binary was not compiled to use: AVX2 FMA
2019-11-25 01:41:07.702208: I tensorflow/core/platform/profile_utils/cpu_utils.cc:94] CPU Frequency: 2904000000 Hz
2019-11-25 01:41:07.702829: I tensorflow/compiler/xla/service/service.cc:168] XLA service 0x56002614df70 executing computations on platform Host. Devices:
2019-11-25 01:41:07.702853: I tensorflow/compiler/xla/service/service.cc:175] StreamExecutor device (0): <undefined>, <undefined>
W1125 01:41:07.708853 139694476597056 deprecation_wrapper.py:119] From /var/tf_cnn/cnn-example.py:95: The name tf.summary.FileWriter is deprecated. Please use tf.compat.v1.summary.FileWriter instead.
W1125 01:41:07.801013 139694476597056 deprecation_wrapper.py:119] From /var/tf_cnn/cnn-example.py:96: The name tf.global_variables_initializer is deprecated. Please use tf.compat.v1.global_variables_initializer instead.
2019-11-25 01:41:07.989450: W tensorflow/compiler/jit/mark_for_compilation_pass.cc:1412] (One-time warning): Not using XLA:CPU for cluster because envvar TF_XLA_FLAGS=--tf_xla_cpu_global_jit was not set. If you want XLA:CPU, either set that envvar, or use experimental_jit_scope to enable XLA:CPU. To confirm that XLA is active, pass --vmodule=xla_compilation_cache=1 (as a proper command-line flag, not via TF_XLA_FLAGS) or set the envvar XLA_FLAGS=--xla_hlo_profile.
2019-11-25 01:41:08.168385: W tensorflow/core/framework/allocator.cc:107] Allocation of 33554432 exceeds 10% of system memory.
2019-11-25 01:41:08.378643: W tensorflow/core/framework/allocator.cc:107] Allocation of 33554432 exceeds 10% of system memory.
2019-11-25 01:41:08.684933: W tensorflow/core/framework/allocator.cc:107] Allocation of 33554432 exceeds 10% of system memory.
2019-11-25 01:41:09.166204: W tensorflow/core/framework/allocator.cc:107] Allocation of 33554432 exceeds 10% of system memory.
2019-11-25 01:41:09.250751: W tensorflow/core/framework/allocator.cc:107] Allocation of 33554432 exceeds 10% of system memory.
Epoch: 0, Accuracy: 0.132812, Loss: 161.859375
Epoch: 100, Accuracy: 0.171875, Loss: 2.229168
Epoch: 200, Accuracy: 0.312500, Loss: 1.879733
Epoch: 300, Accuracy: 0.265625, Loss: 2.301287
Epoch: 400, Accuracy: 0.453125, Loss: 1.666916
Epoch: 500, Accuracy: 0.414062, Loss: 1.660650
Epoch: 600, Accuracy: 0.421875, Loss: 1.392702
Epoch: 700, Accuracy: 0.609375, Loss: 1.102491
Epoch: 800, Accuracy: 0.640625, Loss: 1.099843
Epoch: 900, Accuracy: 0.593750, Loss: 1.259872
Test Accuracy: 0.541300
실질적으로 Code에 작성한 내용이 적용된 것을 확인할 수 있다.
tfjob-cnn-worker-1을 살펴보면 다음과 같다.
$ kubectl logs -n kubeflow tfjob-cnn-worker-1
WARNING: Logging before flag parsing goes to stderr.
W1125 01:37:11.782354 140332716521280 deprecation_wrapper.py:119] From /var/tf_cnn/cnn-example.py:73: The name tf.placeholder is deprecated. Please use tf.compat.v1.placeholder instead.
Downloading data from https://www.cs.toronto.edu/~kriz/cifar-10-python.tar.gz
170500096/170498071 [==============================] - 227s 1us/step
170508288/170498071 [==============================] - 227s 1us/step
W1125 01:41:03.455419 140332716521280 deprecation_wrapper.py:119] From /var/tf_cnn/cnn-example.py:33: The name tf.truncated_normal is deprecated. Please use tf.random.truncated_normal instead.
W1125 01:41:03.465255 140332716521280 deprecation_wrapper.py:119] From /var/tf_cnn/cnn-example.py:37: The name tf.nn.max_pool is deprecated. Please use tf.nn.max_pool2d instead.
W1125 01:41:03.524669 140332716521280 deprecation.py:506] From /var/tf_cnn/cnn-example.py:64: calling dropout (from tensorflow.python.ops.nn_ops) with keep_prob is deprecated and will be removed in a future version.
Instructions for updating:
Please use `rate` instead of `keep_prob`. Rate should be set to `rate = 1 - keep_prob`.
W1125 01:41:03.547966 140332716521280 deprecation.py:323] From /var/tf_cnn/cnn-example.py:84: softmax_cross_entropy_with_logits (from tensorflow.python.ops.nn_ops) is deprecated and will be removed in a future version.
Instructions for updating:
Future major versions of TensorFlow will allow gradients to flow
into the labels input on backprop by default.
See `tf.nn.softmax_cross_entropy_with_logits_v2`.
W1125 01:41:03.572591 140332716521280 deprecation_wrapper.py:119] From /var/tf_cnn/cnn-example.py:85: The name tf.train.RMSPropOptimizer is deprecated. Please use tf.compat.v1.train.RMSPropOptimizer instead.
W1125 01:41:03.719360 140332716521280 deprecation.py:506] From /usr/local/lib/python2.7/dist-packages/tensorflow/python/training/rmsprop.py:119: calling __init__ (from tensorflow.python.ops.init_ops) with dtype is deprecated and will be removed in a future version.
Instructions for updating:
Call initializer instance with the dtype argument instead of passing it to the constructor
W1125 01:41:03.896722 140332716521280 deprecation_wrapper.py:119] From /var/tf_cnn/cnn-example.py:89: The name tf.summary.scalar is deprecated. Please use tf.compat.v1.summary.scalar instead.
W1125 01:41:03.900244 140332716521280 deprecation_wrapper.py:119] From /var/tf_cnn/cnn-example.py:92: The name tf.summary.merge_all is deprecated. Please use tf.compat.v1.summary.merge_all instead.
W1125 01:41:03.901213 140332716521280 deprecation_wrapper.py:119] From /var/tf_cnn/cnn-example.py:94: The name tf.Session is deprecated. Please use tf.compat.v1.Session instead.
2019-11-25 01:41:03.902050: I tensorflow/core/platform/cpu_feature_guard.cc:142] Your CPU supports instructions that this TensorFlow binary was not compiled to use: AVX2 FMA
2019-11-25 01:41:03.915105: I tensorflow/core/platform/profile_utils/cpu_utils.cc:94] CPU Frequency: 2904000000 Hz
2019-11-25 01:41:03.915844: I tensorflow/compiler/xla/service/service.cc:168] XLA service 0x55ffa4901170 executing computations on platform Host. Devices:
2019-11-25 01:41:03.915865: I tensorflow/compiler/xla/service/service.cc:175] StreamExecutor device (0): <undefined>, <undefined>
W1125 01:41:03.917720 140332716521280 deprecation_wrapper.py:119] From /var/tf_cnn/cnn-example.py:95: The name tf.summary.FileWriter is deprecated. Please use tf.compat.v1.summary.FileWriter instead.
W1125 01:41:03.994302 140332716521280 deprecation_wrapper.py:119] From /var/tf_cnn/cnn-example.py:96: The name tf.global_variables_initializer is deprecated. Please use tf.compat.v1.global_variables_initializer instead.
2019-11-25 01:41:04.170462: W tensorflow/compiler/jit/mark_for_compilation_pass.cc:1412] (One-time warning): Not using XLA:CPU for cluster because envvar TF_XLA_FLAGS=--tf_xla_cpu_global_jit was not set. If you want XLA:CPU, either set that envvar, or use experimental_jit_scope to enable XLA:CPU. To confirm that XLA is active, pass --vmodule=xla_compilation_cache=1 (as a proper command-line flag, not via TF_XLA_FLAGS) or set the envvar XLA_FLAGS=--xla_hlo_profile.
2019-11-25 01:41:04.290627: W tensorflow/core/framework/allocator.cc:107] Allocation of 33554432 exceeds 10% of system memory.
2019-11-25 01:41:04.446182: W tensorflow/core/framework/allocator.cc:107] Allocation of 33554432 exceeds 10% of system memory.
2019-11-25 01:41:04.669048: W tensorflow/core/framework/allocator.cc:107] Allocation of 33554432 exceeds 10% of system memory.
2019-11-25 01:41:04.880516: W tensorflow/core/framework/allocator.cc:107] Allocation of 24576000 exceeds 10% of system memory.
2019-11-25 01:41:04.880522: W tensorflow/core/framework/allocator.cc:107] Allocation of 24576000 exceeds 10% of system memory.
Epoch: 0, Accuracy: 0.140625, Loss: 149.115295
Epoch: 100, Accuracy: 0.164062, Loss: 2.234350
Epoch: 200, Accuracy: 0.210938, Loss: 2.128623
Epoch: 300, Accuracy: 0.234375, Loss: 1.992562
Epoch: 400, Accuracy: 0.437500, Loss: 1.613274
Epoch: 500, Accuracy: 0.296875, Loss: 1.885946
Epoch: 600, Accuracy: 0.453125, Loss: 1.597952
Epoch: 700, Accuracy: 0.546875, Loss: 1.433665
Epoch: 800, Accuracy: 0.546875, Loss: 1.271549
Epoch: 900, Accuracy: 0.507812, Loss: 1.446969
Test Accuracy: 0.538000
2개의 결과가 다른것을 확인할 수 있고 이로 인하여 각각의 Pod에서 서로 독립적인 Trainning을 진행한 것을 알 수 있다.
모든 Trainning이 진행되고 Pod 이 Completed가 된 것을 확인하면 지정한 PV의 Mount Path에 각각의 Tensorflow Events가 생기는 것을 확인할 수 있다.
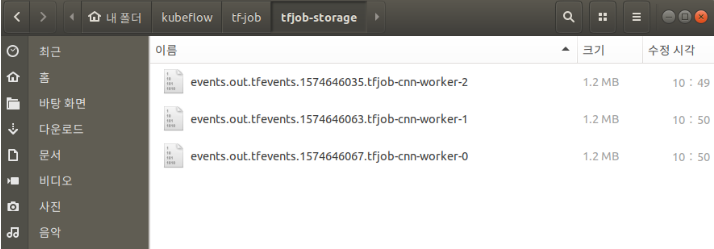
결과인 Events.out.tfevents 또한 Tensorboard에 접속하여 확인 가능하다.
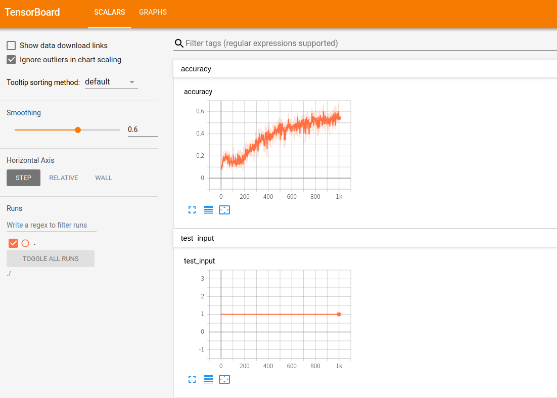
참조:TF-JOB
코드에 문제가 있거나 궁금한 점이 있으면 wjddyd66@naver.com으로 Mail을 남겨주세요.
Leave a comment